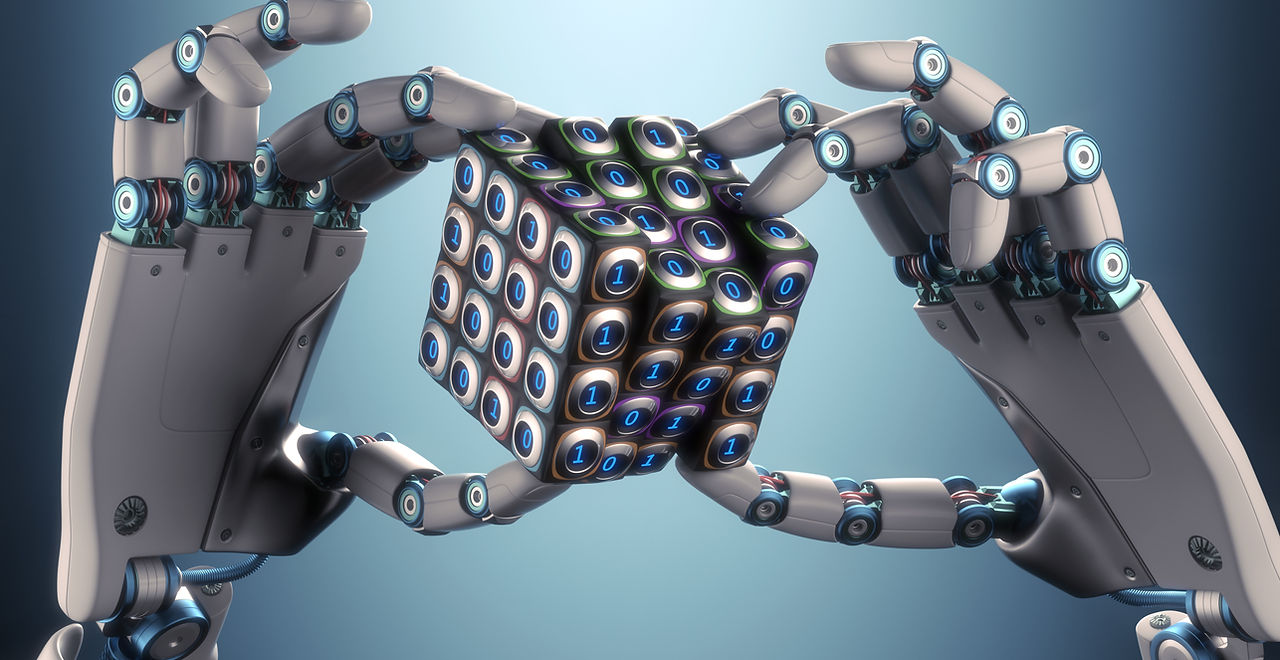
THE RISE OF THE MACHINES?
In James Cameron’s sci-fi classic, The Terminator (1984), Kyle Reese famously warns Sarah Connor, “A few years from now… defense network computers... new… powerful… hooked into everything, trusted to run it all. They say it got smart; a new order of intelligence. Then it saw all people as a threat, not just the ones on the other side. Decided our fate in a microsecond: extermination.” Reese, a future-soldier in the war against those machines, is describing the fate of mankind in the year 2029. This apocalyptic notion likely seemed far-fetched to audiences in 1984, when conventional computers were limited to archaic word-processors and merely owning a computer was deemed a “hobby” reserved for the few “nerds” and “geeks” who understood how to use them. Despite the widespread popularity of smartphones today (which place exponentially more processing power in our pockets than the best computers of 1984 ever did), the thought of true artificial intelligence – a computer capable of learning and thinking on its own – is still considered fiction by many… or a distant fantasy at best. However, in light of recent insight into how we process and learn new information, perhaps this “fiction” isn’t far from becoming a reality.
Within the last decade alone, we have witnessed voice recognition technology transform from a glitch-ridden novelty into something helpful, reliable, and integral to daily life. This is all thanks to personal assistant apps like Alexa, Siri, and Google Assistant. As intuitively as these apps may help us navigate across town, find the perfect dinner reservation, or keep our schedules in order, it’s easy to forget we’re still dealing with very limited technology. Despite efforts to personify these applications by giving them names and human-like voices, they only respond to a predetermined script – merely “parroting” responses to specific prompts. The missing piece (and largest hurdle) to remedying this has been in achieving artificial intelligence that possesses the ability to recognize connections between similar events, apply relevant information/experience/context, and adapt accordingly under its own initiative (aka “learning,” as opposed to “mimicking”).
According to Ray Kurzweil, the man Bill Gates refers to as “the best person I know at predicting the future of artificial intelligence,” we will see a dramatic shift in the way we interact with technology sooner than most people think. Kurzweil serves as Google’s current Director of Engineering and holds 26 U.S. patents (including the first flatbed scanner, the first print-to-speech reading machine for the blind, and the first music synthesizer capable of recreating a grand piano as well as other orchestral instruments). In Transcendent Man (2009), a documentary about the life’s work and predictions of Kurzweil (derived from his 2006 book, The Singularity Is Near), Kurzweil discusses The Singularity; the concept that computational technology grows at an inevitably exponential rate because it relies upon the latest available technology to advance. Thus, he suggests that sometime in the 21st century, the simultaneous progress occurring in artificial intelligence, genetics, nanotechnology, and robotics will lead to the creation of a human-machine civilization. Similarly, this is why one wouldn’t necessarily notice a drastic leap in technology from 1950 to 1970, yet present-day technology becomes outdated in a matter of months. Microchips readily become smaller and smaller as their processing power doubles. This is the Law of Accelerating Returns. The continuation of this trend suggests that, eventually, artificial intelligence will progress so quickly that we (as humans) won’t be able to keep up – finding ourselves “left behind” by our own technology as it becomes capable of building and creating new iterations of itself better and faster than we can – ultimately requiring mankind to merge with machines to remain relevant. Kurzweil has even put a date on when he believes this will occur: the year 2029 – shockingly identical to Cameron’s guess in The Terminator.
In a December 2015 article by Science, researchers Brenden Lake (NYU), Ruslan Salakhutdinov (The University of Toronto), and Joshua Tenenbaum (MIT’s Department of Brain and Cognitive Science and the Center for Brains, Minds, & Machines) took a further step in revolutionizing artificial intelligence. Since the 1990s, Salakhutdinov’s team has been exploring ways to further understand the way we learn, using these findings to create deep neural network algorithms that allow computers to learn in a fashion more likened to humans. “It has been very difficult to build machines that require as little data as humans when learning a new concept,” admits Salakhutdinov. “Replicating these abilities is an exciting area of research connecting machine learning, statistics, computer vision, and cognitive science.”
10 years ago, this required Salakhutdinov and his team to rely on vigorous repetition; entering an exhaustive 60,000 training examples into an algorithm which a computer could use to visually recognize hand-written samples of the numbers 1-9. However, Salakhutdinov and his team then made a breakthrough – a new algorithm that dramatically reduces the amount of time required to “teach” the computer these concepts. Furthermore, perhaps the most surprising development is the fact that Salakhutdinov’s new algorithm – once enacted – allows the computer to learn and make associations between concepts on its own, requiring no outside help from humans. This time, instead of recognizing the numbers 1-9, the authors applied their model to “over 1,600 types of handwritten characters in 50 of the world’s writing systems, including Sanskrit, Tibetan, Gujarati, Glagolitic – and even invented characters such as those from the television series Futurama.” When these computer-generated characters were shown to human participants, fewer than 25 percent were able to decipher which characters were written by a human vs. the computer, further skewing the lines between man and machine.
While the ultimate outcome of these rapid advancements remains uncertain, the evidence suggests we’re closer to “the world of the future” than previously thought. “Before they get to kindergarten, children learn to recognize new concepts from just a single example, and can even imagine new examples they haven’t seen,” notes Tenenbaum. “I’ve wanted to build models of these remarkable abilities since my own doctoral work in the late nineties. We are still far from building machines as smart as a human child, but this is the first time we have had a machine able to learn and use a large class of real-world concepts – even simple visual concepts such as handwritten characters – in ways that are hard to tell apart from humans.” Could it be possible that we are witnessing a transformational moment in our own history? A moment we will remember as pivotal: a moment when we crossed the threshold and built the first machines that no longer needed us? Is this… the rise of the machines?